- Ark's Newsletter
- Posts
- AI Will Replace These Jobs First: A Warning From OpenAI’s Chief Of Research
AI Will Replace These Jobs First: A Warning From OpenAI’s Chief Of Research
Where we stand today is that if you have a job that can be done remotely, which includes most white-collar, laptop-based work, you might want to improve your skills in that area. This shift seems quite achievable. It’s interesting because technology has historically replaced blue-collar jobs, but now, it’s replacing bureaucratic roles. Meanwhile, blue-collar workers, such as plumbers, remain secure, but professionals like lawyers may be more at risk.

Right now, technology is cutting out the mundane aspects of many jobs. For example, tasks like writing complex configurations used to be laborious, but now tools like GPT can handle these. However, human intelligence is still needed to guide more complex or creative tasks. This raises questions about the trajectory of artificial general intelligence (AGI) and how far we are from reaching the point where machines can do everything humans can. The path to AGI will likely bring unpredictable developments, and while we may not have “Westworld”-like robots soon, we are seeing advancements that will continue to redefine job roles.
A key open problem is unlocking creativity in AI systems. In structured environments like games, AI has demonstrated creativity, but figuring out how to allow it to be creative in the real world remains a challenge. Current models, like GPT, mimic human actions, but true creativity requires more complex problem-solving capabilities. As researchers, the focus is on creating environments, such as programming tools, that AI can use to experiment and learn from feedback.

Looking at the next 10 to 20 years, there is still much progress to be made. While current GPT models can accomplish impressive feats, achieving human-level intelligence will involve overcoming many more hurdles. Even as these models grow in scale, the gap between human intelligence and artificial intelligence may not close as fast as we imagine, as every leap in capability demands new innovations.
The journey toward artificial general intelligence (AGI) is still fraught with uncertainty. One of the big challenges lies in automating not just repetitive tasks but also unlocking true creativity in machines. In structured environments like games, AI has proven capable of innovation, but the real world is much more complex. Researchers are trying to develop AI that can operate creatively within the “structured environment” of human reality, yet this presents major obstacles. Currently, environments such as coding and interpreting are where AI excels, as they offer feedback loops that allow the system to learn and improve dynamically.
Interestingly, while 3D environments for training robots seem promising, they are limited because they require manually creating every interaction and object within them. Instead, AI finds more meaningful learning experiences in dynamic, real-world applications like coding, where it can continually test, write, and refine its outputs. The next frontier might involve teaching AI how to simulate and engage with complex environments that mirror human experiences more closely.
A significant point to note is how human feedback and reinforcement learning have shaped the development of models like GPT-4. Despite massive pre-training on internet data, the crucial final steps involve fine-tuning with human input, which helps the models approach tasks in a way that feels more intuitive. This combination of machine learning with human oversight has allowed AI to progress, but challenges remain, especially as expectations for AI grow. Some users have reported that AI seems to be performing worse over time, but this could be more about fluctuating expectations and the inherently probabilistic nature of these systems.

As we move toward GPT-5, 6, and beyond, the scale of AI’s abilities will increase, but the ultimate challenge will be reaching human-level intelligence. Despite the impressive advancements, we are still far from matching the sheer complexity of the human brain. Each leap forward requires overcoming new hurdles, and while there may be no definitive limit to how much AI can improve, predicting the timeline remains difficult. The current models might simulate the intelligence of smaller animals like cats, but matching the human brain will require orders of magnitude more growth. In the coming years, breakthroughs in neural network architectures, scaling, and creative problem-solving will determine just how close AI can get to surpassing human intelligence.
As AI continues to scale, the comparison between the computational power of AI models and the human brain comes into focus. While GPT-3 or GPT-4 may have the computational equivalent of smaller animals in terms of “neurons,” reaching the complexity of the human brain will require advancements that go beyond simply increasing the number of parameters or data fed into models.
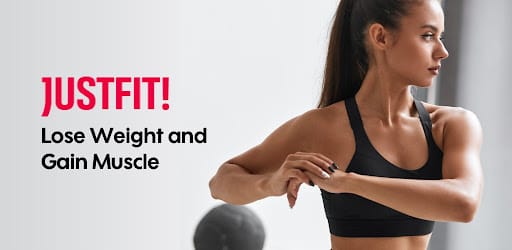
The leap from neural networks and current models to true AGI is not just about size. Each new breakthrough—such as the introduction of transformers in AI—brings us closer, but we also face diminishing returns in some areas, requiring more profound innovations. For example, while previous advancements in AI seemed revolutionary (e.g., neural networks compared to linear regression), the improvements today, though significant, are often the result of numerous incremental advancements and optimizations rather than paradigm-shifting breakthroughs.
Looking ahead, AGI development might follow an “S-curve” pattern. Instead of exponential growth leading to an instant “singularity,” there could be various stages, with plateaus that require entirely new approaches or techniques to push past. Each plateau could signal the need for creative solutions to further scale and improve AI systems. Whether it’s the development of self-improving AI (where models can improve without human input) or figuring out how to integrate AI into more nuanced aspects of human cognition, there are still many unknowns.

An interesting concept being explored is AI’s ability to learn autonomously. While current models like GPT-4 rely heavily on human training data, the idea of having future AI models that can learn by themselves in more sophisticated ways is intriguing. The hope is to eventually reach a point where AI can teach itself new concepts and improve iteratively, reducing reliance on human-guided reinforcement learning.
However, as AI progresses toward and beyond human-level intelligence, unforeseen challenges will arise. There may be scenarios where the methods we’ve used to train AI so far, like mimicking human behavior, become inadequate. We might need to invent new methodologies entirely—approaches that go beyond what we currently understand about intelligence and learning. This will require a shift in both thinking and technology, with AI potentially evolving into systems that no longer just replicate human abilities but begin to surpass them in ways we can’t fully predict today.

For now, the future looks promising, with no clear indication of when AI will hit an absolute limit. Researchers anticipate continued progress over the next five to ten years, with AGI potentially reshaping many aspects of society. But even if AGI is achieved, how it interacts with and integrates into human life remains one of the biggest questions of the coming decades.
As AI continues to evolve and approach AGI, the societal and ethical implications become increasingly significant. Once AI surpasses human-level intelligence, it could bring about drastic changes in the workforce, scientific research, and even how we perceive creativity and intelligence itself. Some areas like creative problem-solving, empathy, and emotional intelligence—traits that have been traditionally considered uniquely human—could also be replicated or enhanced by advanced AI systems.

One of the fundamental shifts we’re seeing is in how AI is integrated into everyday work. As mentioned earlier, technology is already automating repetitive, “rote” parts of jobs, but what about tasks that require higher-order thinking? While current models like GPT are adept at performing tasks with defined inputs and outputs, they still rely heavily on human guidance for complex, creative, or novel problems. However, as AI systems learn to operate more autonomously, even those areas might begin to change. The development of self-learning models or AI capable of continuous improvement without human intervention could bring us closer to a future where AI doesn’t just assist but actively drives innovation.
Moreover, once AI reaches or surpasses human-level intelligence, it could fundamentally alter how industries operate. For example, industries that require complex decision-making, such as medicine, law, and even governance, might begin to rely more on AI systems capable of making sophisticated judgments based on vast datasets. This raises questions about trust, accountability, and control—who is responsible for an AI’s decision, and how do we ensure it aligns with human values?

Additionally, the scaling up of AI could lead to a scenario where it helps to solve global challenges that humans have struggled with, such as climate change, poverty, or resource management. The ability to process enormous amounts of data and simulate outcomes in real time could make AI indispensable in fields like environmental science, economics, and geopolitics. However, this also introduces new risks—AI systems that are misaligned with human goals or misused could lead to unintended consequences, from economic instability to ethical dilemmas regarding privacy and autonomy.
As AI systems grow more advanced, researchers also speculate about the potential for AI to develop entirely new forms of intelligence—ways of thinking and problem-solving that are not bound by human cognitive limitations. This could lead to breakthroughs in areas we currently cannot even imagine. At the same time, it brings a level of unpredictability to the table. Once AI systems are capable of self-improvement, their evolution could accelerate beyond human control, raising concerns about how we manage and oversee these systems to ensure they remain aligned with human welfare.

In conclusion, the trajectory of AI development points to continued rapid advancements, with major implications for society, the workforce, and global challenges. While AGI could potentially offer solutions to some of humanity’s most pressing problems, it also presents risks that need to be carefully managed. The road to AGI will involve not just technical breakthroughs but also ethical, social, and governance challenges that will need to be addressed in the coming decades.

